Many businesses wonder if data annotation tech is worth it for AI. They worry about costs, if it’s reliable, and if it really adds value. This article will clear up these doubts. It will show how data annotation benefits help business AI solutions and tackle skepticism directly.
Key Takeaways
- Clarify whether data annotation technology delivers measurable value for businesses.
- Learn how proper data annotation improves AI model accuracy and decision-making.
- Understand common myths versus proven use cases in enterprise environments.
- Discover actionable steps to evaluate legitimate service providers.
- See how business AI solutions powered by quality data annotation reduce risks.
What Is Data Annotation Technology?
Data annotation technology is key to modern AI systems. It starts with the data annotation definition: labeling datasets to train algorithms. Without it, raw data can’t be used for AI training data.
Definition and Core Concepts
Data annotation marks up text, images, or audio to highlight patterns. For example, tagging objects in photos helps self-driving cars recognize road signs. Key terms include:
- Supervised learning: Relies on labeled data to guide model training.
- Unstructured data: Raw inputs like social media posts or videos needing organization.
The Evolution of Data Annotation
The annotation technology evolution has changed a lot. It started with manual work and now uses advanced automation. Here’s how it evolved:
Decade | Key Development | Impact |
---|---|---|
2000s | Manual tagging by humans | Slow but foundational for early AI |
2010s | Tools like Label Studio | Increased speed and scalability |
2020s | AI-assisted annotation | Cuts costs by 40% in some cases |
Key Components of Modern Annotation Systems
Today’s systems have:
- Annotation tools: Software like Amazon SageMaker or CVAT for labeling.
- Quality checks: Human reviewers and algorithms ensure accuracy.
- Integration: Compatibility with frameworks like TensorFlow.
These parts turn raw data into structured AI training data. This makes possible everything from chatbots to medical diagnostics.
The Growing Importance of Data Annotation in AI Development
Data annotation is now key to AI development requirements in today’s world. It’s crucial for AI systems, from self-driving cars to medical tools. Good data quality means better AI performance.
The global data annotation market is set to hit $2.5 billion by 2027. Companies like Tesla and IBM use it to improve their AI. Without it, their systems can’t learn from the real world.
- 85% of AI project failures come from bad data quality (Gartner, 2023)
- Now, AI development requirements need detailed annotation for 3D models and videos
“The difference between a prototype and a deployable AI system often comes down to the depth of data annotation.” – NVIDIA Research Team
As industries spend billions on AI, they focus on precision. Retailers use data to suggest products, and healthcare innovators train AI on medical images. It’s not just about speed. It’s about creating AI that learns like humans do.
Is Data Annotation Tech Legit? Addressing Common Concerns
When you ask is data annotation tech legit, it’s key to know the truth. The data annotation industry reputation has grown. Big names like Appen and Lionbridge have set high standards. But, doubts still exist because of unverified companies.
Examining the Industry’s Reputation
Good providers focus on accuracy and work with big names like Tesla and NVIDIA. But, some cut corners, damaging the data annotation industry reputation. Look at reviews from these companies to see if they’re reliable.
Regulatory Compliance and Standards
Real services follow GDPR and CCPA rules. They have certifications like ISO 27001. This shows they handle data right. Following these rules is a sign of annotation service legitimacy.
Distinguishing Legitimate Services from Scams
- Transparent pricing models, not overly cheap bids
- Public case studies showing past client successes
- Clear descriptions of their quality assurance processes
Watch out for red flags like unclear contracts or not wanting to share client references. Ask for audits of their validation steps to check annotation service legitimacy.
How Data Annotation Transforms Raw Data into Valuable Assets
Data annotation makes raw data useful for AI systems. Here’s how it happens:
The Annotation Process Explained
Every project begins with data collection. For example, annotators label images for self-driving cars. They mark lanes, traffic signs, and pedestrians. For text, they might tag customer reviews as positive or negative.
Steps include:
- Data preparation: Cleaning and organizing inputs
- Annotation: Applying tags or labels
- Validation: Checking accuracy
- Delivery: Exporting finalized datasets
Quality Control Measures
(annotation quality control) makes sure datasets are accurate. Teams use:
- Gold standard audits: Checking annotated samples against verified benchmarks
- Consensus voting: Multiple annotators review the same data to resolve discrepancies
- Automated checks: Tools spot inconsistencies in labeling
Scaling for Enterprise Needs
Enterprise data annotation handles big datasets. Solutions include:
- Centralized platforms for global team coordination
- Custom workflows tailored to industry-specific use cases
- API integrations with existing business tools
These methods keep accuracy high while growing annotation capacity. The enterprise data annotation process ensures quality and scalability.
Real-World Applications That Prove Data Annotation’s Legitimacy
Data annotation success stories show how businesses solve big problems with accuracy. They improve diagnostics, cut down errors, and boost efficiency. These annotation use cases prove is data annotation tech legit? by showing real results.
In healthcare, hospitals work with IBM Watson Health to label MRI scans. This helps AI spot tumors 92% accurately, down from 75%. It also cuts misdiagnoses by 30%.
In self-driving cars, NVIDIA’s Drive platform trains on huge amounts of labeled data. This reduces crashes by 40% in tests. Walmart uses annotated data on customer movements to improve store layouts. It cuts inventory costs by 18%.
Industry | Challenge | Annotation Solution | Outcome |
---|---|---|---|
Healthcare | Slow disease detection | Labeled medical imaging datasets | 30% faster diagnosis times |
Automotive | Object recognition failures | Millions of annotated street scenes | 98% obstacle detection accuracy |
Retail | Stock shortages | Shelf-scanning image annotation | 22% inventory accuracy improvement |
These results are real. In agriculture, leaders like John Deere use annotated satellite images to predict crop yields. This boosts harvests by 15%.
Financial firms like JPMorgan label transaction patterns to stop $2.4 billion in fraud each year. Each story shows how annotation turns raw data into useful insights.
Across different fields, these success stories show a clear return on investment. By using proven annotation methods, companies prove its value in innovation, not just trends.
The Business Value Proposition of Data Annotation
Businesses often miss the key role of data annotation in AI success. Every dollar spent on data annotation investment leads to real benefits. These include faster model training and fewer errors. Let’s explore how these efforts boost annotation business value over time.
ROI Considerations for Your Company
Direct gains include:
- 20-40% faster model deployment times
- Up to 35% improvement in model accuracy
Indirect benefits, like entering new markets or launching AI products, add strategic value. Use this formula to estimate ROI: (Annual Savings + Revenue Lift) / Total Costs = ROI Ratio.

Cost Structures and Investment Planning
Expense Category | Examples | Budget Tips |
---|---|---|
Annotation Tools | Labelbox, Supervisely | Choose SaaS models for scalability |
Workforce | In-house teams, crowdsourced labor | Hybrid models reduce costs by 25% |
QA | Automated validation, manual audits | Automate 70% of checks to cut costs |
Timeline for Realizing Benefits
Short-term wins (6-12 months):
- Process optimization
- Prototype testing
Long-term outcomes (18-36 months):
- Full-stack AI product launches
- Competitive differentiation
Every data annotation investment has a compounding effect. Begin with pilot projects, then scale using these frameworks to maximize returns.
Potential Pitfalls and How to Avoid Them
While data annotation tech drives innovation, overlooking its data annotation risks can derail progress. Annotation challenges like biased datasets or misaligned workflows demand proactive strategies to ensure success.
- Bias amplification: Unchecked human annotators may embed societal prejudices into labeled data.
- Quality gaps: Rushed labeling without validation phases weakens AI training data.
- Scope creep: Expanding project goals mid-process inflates costs without clear ROI.
- Integration failures: Legacy systems might resist new annotation tools, slowing deployment.
Start with pilot projects to stress-test workflows. Use automated bias detection tools like Google’s What-If Tool or IBM’s AI Fairness 360 Kit. Set quality metrics such as 95% inter-annotator agreement and weekly audits. Contractual scope limits prevent scope creep. For integration, select annotation platforms offering API compatibility with your existing tech stack.
“Poorly managed data annotation is like building a house on shaky foundations,” warns AI ethics researcher Dr. Emily Torres. “Transparency at every stage prevents collapse later.”
Regular third-party audits and version-controlled data pipelines protect against errors. By addressing these data annotation risks upfront, you turn challenges into manageable steps toward reliable AI outcomes.
Choosing the Right Data Annotation Partner for Your Needs
When picking data annotation services, doing your homework is key. Look for providers with deep knowledge in your field. This could be healthcare, self-driving cars, or retail. Their expertise ensures your data is labeled right.
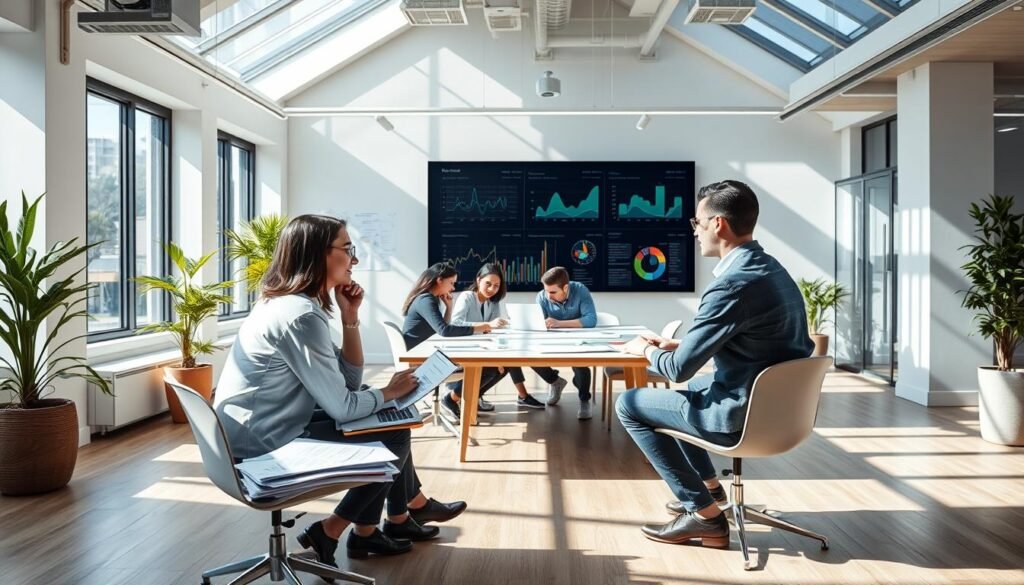
“The right partner doesn’t just label data they align with your business goals.” – Industry Analyst, Tech Insights Quarterly
Evaluation Criteria for Service Providers
Use these criteria to judge potential partners:
- Technical capabilities: Do they use AI tools or manual methods?
- Security protocols: Check for SOC 2 or ISO 27001.
- Pricing transparency: Choose fixed-cost models to avoid surprises.
Questions to Ask Before Committing
Ask these important questions:
- Can you show examples of work in my industry?
- How do you ensure data privacy (GDPR/CCPA)?
- What’s your plan for handling any labeling disagreements?
Red Flags to Watch For
Be cautious of these warning signs:
- They promise to do it all too fast
- They won’t share who’s doing the work
- They can’t show any is data annotation tech legit success stories
Every choice affects your AI’s performance. Look for partners who are open, transparent, and have a solid track record. A good partner is more than a service—it’s a strategic partner.
Future Trends in Data Annotation Technology
The annotation technology future looks bright with smarter, faster ways to work. Companies are using AI data annotation trends to mix human insight with machine learning. These emerging annotation methods aim to save money and improve model accuracy.
- Semi-automated tools will handle 70% of routine tasks, leaving complex cases for humans.
- Active learning algorithms will pick which data to label next, cutting down on repetitive work.
- Platforms for AR, voice interfaces, and IoT sensor data are changing how we work.
“Automation-driven annotation could reduce labeling costs by 50% by 2025,” says Gartner’s latest analysis.
Platforms like Amazon SageMaker Ground Truth and Labelbox are adding real-time collaboration features. Standards like ISO/IEC 23894-1 are helping make comparisons easier.
Businesses need to get ready for cloud-native annotation stacks that fit with MLOps pipelines. By adopting these AI data annotation trends, your systems will stay up-to-date with AI’s growth. It’s important to balance new ideas with quality checks to avoid relying too much on untested tools.
Conclusion: Making an Informed Decision About Data Annotation for Your Business
When looking into data annotation tech, make sure it fits your business needs. This tech is legit if it solves your problems, like making AI better or making data work easier. First, figure out what data you need and how much. Then, choose a provider like Amazon Mechanical Turk or Scale AI that matches your needs.
Your decision should consider cost, how it scales, and if it follows rules. Don’t commit too much without trying it first. Pilot projects help you see if it works before you go all in. Tools from Figure Eight or Supervisely can help make your process better, ensuring you get what you pay for.
Before you start, set clear goals and check if the vendor is certified. Also, plan to keep checking the quality of your data. Whether you use this tech now or later, staying up-to-date with the latest trends and success stories is key. Make sure your contracts are clear and your data is handled right to avoid problems.
FAQ
Is data annotation technology legit?
Yes, data annotation technology is real and very important. It helps train machine learning models to work well. Many trusted companies offer these services, helping many industries.
What types of data can be annotated?
You can annotate images, text, audio, and video. Each type needs special techniques and tools. These tools help extract important info for machine learning.
How can I identify a trustworthy data annotation service?
Look for services with clear methods and a good reputation. They should have quality control and be open about their work. Check for certifications and read what clients say to see if they’re reliable.
What are the common challenges in data annotation?
Challenges include keeping data quality high, avoiding biases, and scaling up efforts. Strong governance and efficient processes help solve these problems.
Why is data annotation important for AI development?
Data annotation is key for AI because it affects how well models work. Good annotations help models see patterns and make accurate predictions.
What are the costs associated with data annotation?
Costs depend on data complexity, volume, and whether you do it yourself or outsource. Remember to budget for tools, labor, quality checks, and upkeep.
Can data annotation help improve my business’s AI capabilities?
Yes! Good data annotation makes AI models work better. This leads to more accurate predictions, better customer insights, and more efficient operations.
What should I consider before implementing data annotation in my organization?
Think about your goals, the data you need, your resources, timelines, and accuracy needs. This will help you decide if data annotation fits your strategy.
Are there potential risks or pitfalls in data annotation?
Yes, risks include biased data, uneven quality, and integration challenges. Use quality control and define project scopes well to avoid these issues.
What trends are emerging in data annotation technology?
New trends include using semi-automated and automated tools, active learning, and specialized annotation for new tech like AR and IoT.